S&DS
S&DS
, Yale University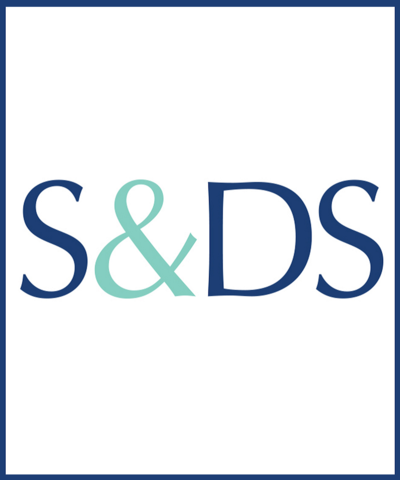
Many Yale faculty will describe data science research opportunities in their research groups.
Statistics & Data Science Project Pitch
January 14, 2019 | 3:45PM to 5:00PM @ Yale Institute for Network Science
Introduction:
Daniel A. Spielman
Department Chair, Statistics and Data Science; Sterling Professor of Computer Science; Professor of Mathematics
Project Pitches:
Damon Clark
Associate Professor, Department of Molecular, Cellular, and Developmental Biology
https://clarklab.yale.edu
damon.clark@yale.edu
Understanding neural circuits that detect visual motion
Claire Bowern
Professor, Director of Graduate Studies
Chair, Yale Women Faculty Forum
Department of Linguistics
http://campuspress.yale.edu/clairebowern/
claire.bowern@yale.edu
Geography and Speech: How Languages Change in Time and Space
All languages are constantly changing. This project looks at untangling language change by investigating change in different accents across North America. Regional accents are salient feature of American speech, but traditional ways of studying accents make it hard to quantify how much variation in American English is conditioned by region, versus other factors that are also known to influence how people speak (such as gender, age, and ethnicity). Using a previously collected geotagged speech sample from about 4000 Americans, this project will examine: 1) how much variation in American English is explained by geography, age, gender, and ethnicity; 2) what features of speech are particularly strong markers of regional dialects; 3) whether there are other aspects of speech which also vary by region which we would predict to vary systematically, but which have not been studied; 4) whether the historical dialect regions identified in prior literature are still maintained in the speech of young adults today. This is one project in a larger research lab on quantitative approaches to language and language change.
Joshua Kalla
Assistant Professor of Political Science and Data Science
http://joshuakalla.com
josh.kalla@yale.edu
Predicting policy learning and adoption after randomized field experiments
The United States spends more per student on K-12 education than other developed countries, yet suffers from poor results. Students in countries as varied as Canada, China, Estonia, Germany, Finland, the Netherlands, New Zealand and Singapore consistently outrank their US counterparts on math and reading ability. To improve the quality of K-12 education, researchers from fields such as statistics, economics, psychology, and education have conducted nearly 200 randomized field experiments to determine which interventions--such as financial incentives for good grades, high-dosage tutoring, and teacher professional development--are most effective at improving student learning and human capital. Despite this research, many school districts fail to adopt policies and programs that have been provably shown to work. In this project, we aim to measure the rate at which successful education interventions are adopted and construct predictive models of which types of schools adopt effective interventions following the publication of an experiment while others do not. Through this research, we can better inform policymakers' ability to use rigorous, evidence-based research to improve K-12 education.
Eduardo Fernandez-Duque
Professor Department of Anthropology and School of Forestry & Environmental Studies
https://fernandezduque.wordpress.com
eduardo.fernandez-duque@yale.edu
Presented by Margaret Corley (Postdoc, Fernandez-Duque Lab)
margaret.corley@yale.edu
Life-history and behavioral consequences of competition between solitary and pair-bonded owl monkeys
The Owl Monkey Project (OMP) of Argentina is an international research program focused on understanding the proximate mechanisms, function, and adaptive value of pair-bonding, monogamy, and biparental care. We are currently utilizing genetic, demographic, and behavioral data from a population of owl monkeys (Aotus azarae) to evaluate the influence that the competition between solitary floaters and resident reproducing adults has on a pair-bonded primate society. Potential projects involving these data include (but are not limited to) 1) using models to assess how survivorship, age at dispersal, and other factors are associated with within-group relatedness, 2) modeling the interactive effects of sex and relatedness on the amount and type of parental care provided to infants, 3) modeling how life-history characteristics are associated with an individual’s likelihood to form a stable pair-bond and the likelihood of its bond’s persistence, and 4) exploring the effect of sex and various life-history characteristics on the distance traveled during natal dispersal.
Douglas Duhaime
Digital Humanities Software Developer, Digital Humanities Laboratory
http://dhlab.yale.edu
douglas.duhaime@yale.edu
Automatic Detection of Image Reuse
While companies like Turnitin.com have built big businesses on the detection of textual plagiarisms, the automatic detection of reused image content remains a difficult problem in machine learning. To help legal scholars and historians better understand the ways statutory and common law copyright regulations have influenced the creation and circulation of visual culture, the Digital Humanities Lab is developing software that identifies full and partial image reuse within massive image datasets. Combining classical image processing techniques (perceptual hashes, SURF, Wasserstein distance) with more recent advancements in convolutional neural networks, this software aims to provide researchers with easy-to-use tools for the study of image similarity across history. This work will involve transforming massive image collections with distributed computing techniques, building ensemble-based machine learning pipelines founded on convolutional neural networks, creating interactive data visualizations of reused image content, and building an open source application that allows non-technical users to study longitudinal trends in the creation and circulation of user-provided image content.
Angel Hsu
Assistant Professor at Yale-NUS College and Adjunct at the Yale School of Forestry and Environmental Studies, Director of Data-Driven Yale, http://www.datadrivenyale.edu, angel.hsu@yale.edu
Presented by Amy Weinfurter (Research Associate at Data-Driven Yale)
amy.weinfurter@yale.edu
Improving algorithms for matching and compiling a global database on non-state and subnational climate change actors
Building predictive models to understand the likelihood of non-state climate commitment implementation
Countries are no longer the sole actors in global climate governance. Cities and regions along with businesses, investors, and civil society organizations play an increasingly prominent role in climate mitigation, adaptation and finance. However, it’s unclear if their efforts can bring the world closer to the global goal of keeping global average temperature increase to “well below” 2°C and “pursue efforts” to limit it below 1.5°C. Understanding their impact is critical, since national government policies are not yet sufficient to meet this global goal, and prevent runaway global warming, on their own. Data-Driven Yale has created the world’s largest database of climate action commitments from cities, regions, companies, investors, and civil society organizations, to help understand the scope and growth of these actors’ participation, the types of actions they have committed to, and their ability to potentially reduce greenhouse gas emissions.
As this database represents an aggregation of a large number of less complete sources, each with different conventions for identifying unique actors, a central challenge in this work lies in harmonising those naming conventions so that unique actors are represented by a single label in our database. This requires (1) matching different names that refer to the same actor (e.g. BMW AG, BMW Group, Bayerische Motoren Werke all refer to the same company), and (2) using other information present in some sources to disambiguate different actors represented by the same name (e.g. London, Ontario; London, Kentucky; and London, England may all be referred to as London but are different actors). Student project(s) would involve creating a matching system scalable to multiple databases with tens of thousands of rows that achieves high performance on both the matching and disambiguating tasks. As a starting point you will have access to a ~25,000 row training/test set of correctly matched names, a script using fuzzy matching algorithms to aid in generating manual correspondences between naming conventions, and preliminary work we have done on incorporating contextual information (e.g., population, revenue, actor type, etc.) into the matching process. Another possible area of research is to help us build new predictive models to understand what factors determine the likelihood of whether these climate action policies get implemented.
Emily Erikson
Associate Professor, Sociology
https://sociology.yale.edu/people/emily-erikson
Emily.erickson@yale.edu
Topic Modeling with Network Methods
Text analysis of a corpus of English economic works from 1580 to 1720 show a shift away from religious and moral works to the topics of trade, finance, and industry. This was a significant turning point for economic thought. A database of 1,308 authors, 6,149 corporate investors, and 304 trade councilors reveals that a large and increasing proportion of texts were authored by merchants who were deeply embedded in networks of corporate investment but occupied peripheral positions in the networks of committees and councils that formed the state’s economic policy branch. The structure of authors’ networks indicates that new economic arguments were developed by merchants attempting to communicate across the divisions between economic and state spheres -- in effect creating a new type of knowledge in order to bridge an existing cultural hole.
Joe Howard
Eugene Higgins Professor of Molecular Biophysics and Biochemistry and Professor of Physics
Co-Director, Quantitative Biology Institute
https://howardlab.yale.edu
jonathon.howard@yale.edu | 2-7245 | Bass Center 334 (enter building through Chemistry)
Development of dendritic branching patterns of nerve cells
We are trying to understand the formation of dendrites, highly branched structures that function as the antennae of neurons, collecting sensory information from the environment or receiving input from other neurons. By imaging cells in living tissue, we have discovered that the dendritic tips of these cells are highly dynamic, converting stochastically between growing, shrinking states. Two feedback mechanisms shape the cells. Positive feedback is mediated by the formation of new branches along extant processes; and negative feedback is mediated by contact-induced retraction whereby a growing tip converts to a shrinking one upon collision with another process. We are characterizing the dynamics of these processes and using them to build mean-field and agent-based models to bridge between the mesoscopic length scales of tips (~0.1 to 1 micron) and the macroscopic length scale of the whole cell (~500 microns). There are several projects involving the analysis of this branching process.
Dirk Bergemann
Douglass and Marion Campbell Professor of Economics
Chair, Department of Economics
http://campuspress.yale.edu/dirkbergemann
dirk.bergemann@yale.edu
We study the classic sequential screening problem in the presence of buyers’ ex-post participation constraints. A leading example is the online display advertising market, in which publishers frequently do not use up-front fees and instead use transaction-contingent fees. We establish conditions under which the optimal selling mechanism is static and buyers are not screened with respect to their interim type, or sequential and the buyers are screened with respect to their interim type. In particular, we provide an intuitive necessary and sufficient condition under which the static contract is optimal for general distributions of ex-post values.
Donna Spiegelman
Susan Dwight Bliss Professor of Biostatistics
https://medicine.yale.edu/people/search/donna_spiegelman.profile
donna.spiegelman@yale.edu
Methods for design and analysis in implementation and prevention science
Only 14% of biomedical research is ever translated into practice, and among these, fully 17 years passes, on average, between the time of submission of the research article and eventual implementation. For example, cervical cancer is now uncommon in North America and Western Europe, but is the first or second leading cause of cancer mortality among women in low and middle income countries. Yale School of Public Health's new Center on Methods for Implementation and Prevention Science (CMIPS) is developing and disseminating innovative methodologic approaches to address implementation gaps such as these and improve public health worldwide, strategically selecting the issues that carry the greatest burden and hold the greatest promise for amelioration. Among the topics CMIPS will address include novel study designs to be used for the identification of effective and cost-effective intervention strategies at large scale in resource-constrained settings. These include variants of stepped wedge designs, learn as you go designs, two stage designs, and quasi-experimental designs which utilize and integrate existing vast data resources. Such “big data” include claims data, electronic medical records, surveillance systems, and population surveys, which can be deployed to obtain timely answers to key public health policy and health care questions.