Jing Zhang
Jing Zhang
, Yale University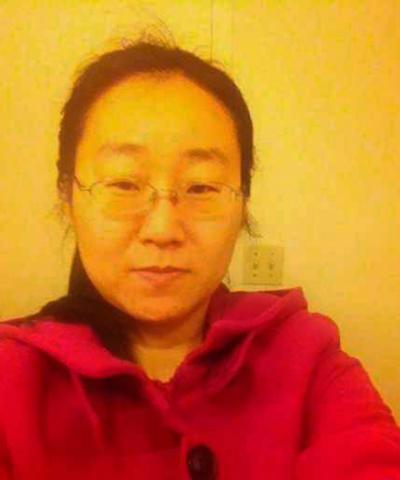
Static pair-wise functional connectivity has been widely used in the neuroimaging field. In contrast, higher-order functional interactions among brain networks and their temporal dynamic transition patterns have been rarely explored. This paper presents a novel dynamic Bayesian variable partition model (DBVPM) that simultaneously considers and models high-order functional interactions and their dynamics via a unified Bayesian framework. Then, we modeled and characterized these temporal state transitions as finite-state machines, and quantitatively compared their transition patterns between post-traumatic stress disorder (PTSD) patients and healthy controls. We found that these interaction patterns are hopping among a finite number of states, and PTSD patients have a different functional interaction state-space and their temporal transition patterns are substantially different in comparison with healthy controls. This work discovered interesting phenomena that cannot be revealed by static pair-wise functional connectivity, thus offering novel opportunities for deciphering the working mechanisms of brain networks in the future.
______________________________________________________________________________________________________________
Multiple Bayesian models equipped with Markov Chain Monte Carlo (MCMC) methods have been recently proposed to search for complicated interactions in the field of Genome-wide association study and HIV drug resistance. Probabilistically modeling mutations in the HIV-1 protease or reverse transcriptase (RT) isolated from drug-treated patients provides a powerful statistical procedure that first detects mutation combinations associated with single or multiple-drug resistance, and then infers detailed dependence structures among the interacting mutations in viral proteins. Combined with molecular dynamics simulations and free energy calculations, Bayesian analysis predictions help to uncover genetic and structural mechanisms in the HIV treatment resistance. Results obtained with such stochastic methods pave the way not only for optimization of the use for existing HIV drugs, but also for the development of the new more efficient antiretroviral medicines. In this talk I will introduce a series of Bayesian partition models proposed in both GWAS and the bioinformatics of anti-HIV therapy, and highlight real applications in GWAS, HIV and HBV studies including HIV drug resistance, cross-resistance, HIV coreceptor usage, and occult HBV infection.