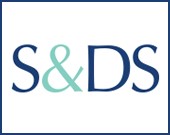
When school let out for summer on Wednesday, close to 100 Yalies left campus with a virtual toolbox in hand — a starter pack of data science methods, excellent for digging into whichever academic fields await them.
These students were enrolled in the first semester of “YData: An Introduction to Data Science,” Yale’s new data science gateway course, co-taught by Jessi Cisewski-Kehe, assistant professor of statistics and data science, and John Lafferty, the John C. Malone Professor of Statistic and Data Science. A few dozen among them also signed up for one of three half-credit seminars, where they applied the skills they’ve learned in YData to text analysis, exoplanet astronomy, or political campaigns.
While some students who took YData intend to major in statistics and data science, many do not. The core class was designed to be accessible and attractive to students with all levels of prior experience and of all academic leanings. In his May 9 update on academic priorities, President Peter Salovey held up YData as an example of Yale’s commitment to offering more multidisciplinary courses and programs.
As dean of social science Alan Gerber explained in a YaleNews preview of the course last semester, YData’s purpose is to “demystify” data science for all Yale students: Neither a humanist nor a scientist should have to go through life seeing the everyday applications of big data and algorithms — from ATMs to Amazon Alexa — as black boxes. Data science is one of five science areas that Yale has recently identified as top priorities for future investment.
English major Liana Van Nostrand ’20 said she decided to take YData this term because she “missed working with numbers.”
“Doing the problem sets for YData feels like working out a part of my brain that has atrophied a bit,” she said. Van Nostrand signed up for Lafferty’s seminar in text analysis because she saw its relevance to journalism, her primary career interest.
Lafferty’s seminar on text analysis attracted a number of humanities-oriented students. He said he’s “really enjoyed working with the students, who have academic interests a bit different from many of the students we more typically have in statistics and data science courses.”
Lafferty began by teaching the “basic but important” computing skills for text analysis. Once the students had a handle on the methods, he had them process books from Project Gutenberg, the free online collection of digitized public domain literature, which includes thousands of well-known classics, from the complete works of Shakespeare to the 19th-century autobiography “Narrative of the Life of Frederick Douglass, An American Slave.”
In February, Lafferty had the class analyze the State of the Union addresses of every U.S. president. “The class wrote code to track the occurrence of keywords over time in the State of the Unions, and tried to see how they matched up with historical context,” said Lafferty, noting that there was even a brand-new address available that week for the students to consider.
The students then applied different machine learning techniques to text, such as using topic models to group scientific articles and movie reviews, and exploring how word embeddings “might encode ‘societal bias’ like gender bias, even when they are constructed on data like Wikipedia,” said Lafferty.
Van Nostrand said that by the end of Lafferty’s class, they had a basic understanding of the text-based algorithms behind applications the average student encounters daily — for example, how Gmail predicts the next words a user will type or how Spotify determines which new songs to suggest.
Joshua Kalla ’14 taught the seminar that explored how contemporary political campaigns use big data and computing to their tactical advantage. Kalla returned to his alma mater as an assistant professor of political science this semester, and this YData seminar was his first class as a Yale instructor.
It was also a return to Kalla’s own academic roots: He first “fell in love” with data-driven political science research in a class he took during his first year at Yale. Taught by Gerber, the Charles C. & Dorathea S. Dilley Professor of Political Science, that gateway course covered new experimental methods in political science research.
Of all the readings on that pivotal course’s syllabus, it was Gerber’s own studies — done in partnership with Donald Green, a political scientist who was at Yale through 2011 — that captivated Kalla’s interest most. Borrowing the methods of clinical trials in medicine, in the early 2000s Gerber and Green were the first to apply the randomized field experiment to American political science research. They began treating age-old political campaign tactics — lawn signs, canvassing, get-out-the-vote mailings — as interventions that, like new drugs, could be tested, measured, and improved.
Kalla used some of this same randomized field research to teach his YData seminar students, asking them to reanalyze data from a study by Gerber and Gregory Huber, the Forst Family Professor of Political Science, where they tested the effectiveness of sending get-out-the-vote postcards printed with a recipient’s name and own voting history plus the voting history of their neighbors.
Nicholas Begotka ’22, one of Kalla’s students, said one of the things he learned in the seminar that surprised him most was the sheer amount of voter data available to political campaigns.
“A lot of your ‘personal’ information is public and accessible to campaigns,” said Begotka. “Voter files can contain up to 103 variables for each potential voter, including your name, address, and voting history, to name a few. The fact that a company could be compiling and selling your information to campaigns at this moment is so crazy to think about.”
Political campaigns’ use of this data does raise serious ethical questions, Kalla explained, which is why he devoted an entire class period to discussing the ethics of personal data use for campaign tactics. For the final project, Kalla asked his students to turn a critical eye on the way political campaigns are using data science today by selecting a recent campaign and analyzing its tactics with special attention to the ethical dimension.
Because of his experiences in both YData and Kalla’s seminar, Begotka said, he wants to learn even more about the intersection between data science and political science.
“I think it would be really cool to learn how to use data in advancing political causes, winning elections, and creating change in politics,” said Begotka. “Whether I decide to major in data science, I’m looking forward to continuing with the subject.”
The seminar on exoplanet astronomy — the most STEM-focused topic of the three — attracted students with more advanced coding skills. This allowed Cisewski-Kehe to have the class jump right into detecting exoplanets using the transit and radial velocity methods, the two most popular approaches for exoplanet detection.
To learn the transit method, the students pulled data from NASA’s Kepler Mission and had to figure out how to align the transits, which are the dips in the light output of the host star suggesting an object, such as a planet, is crossing between the star and the telescope. Using the radial velocity method, the students had to model the shape and speed of the planet’s orbit, Cisewski-Kehe explained.
Cisewski-Kehe said that in teaching both the core course and her seminar, she noticed that students particularly appreciated working with real data examples. To broaden the scope of topics for real data examples, YData will need to offer more seminars in the future, she notes.
“We hope that faculty from around campus in many different disciplines will be interested in designing their own YData seminar,” she said. “Students seem eager to learn about how data science applies to different settings. Each YData seminar topic could look very different, so students might even want to take multiple seminars depending on their academic and personal interests.”
Lafferty added that he’d welcome working with colleagues in the humanities and other areas to help them design and implement a YData seminar on a topic related to their field. “My hope is that such courses will give students a new angle on their academic interests, and that the programming and data analysis skills will help them in their later work,” he said.
For Van Nostrand, at least, Lafferty’s hope was realized.
“As a second semester junior and English major, I think it would be a little late for me to change my major or academic trajectory substantially, but I do want to continue taking data science courses next year,” said Van Nostrand. “I think having some data science skills could be helpful professionally. I’m hoping to enter a career related to journalism, where data science is becoming increasingly important.”